AI Supporting Annual Teaching Report
- Jace Hargis
- 2 days ago
- 3 min read
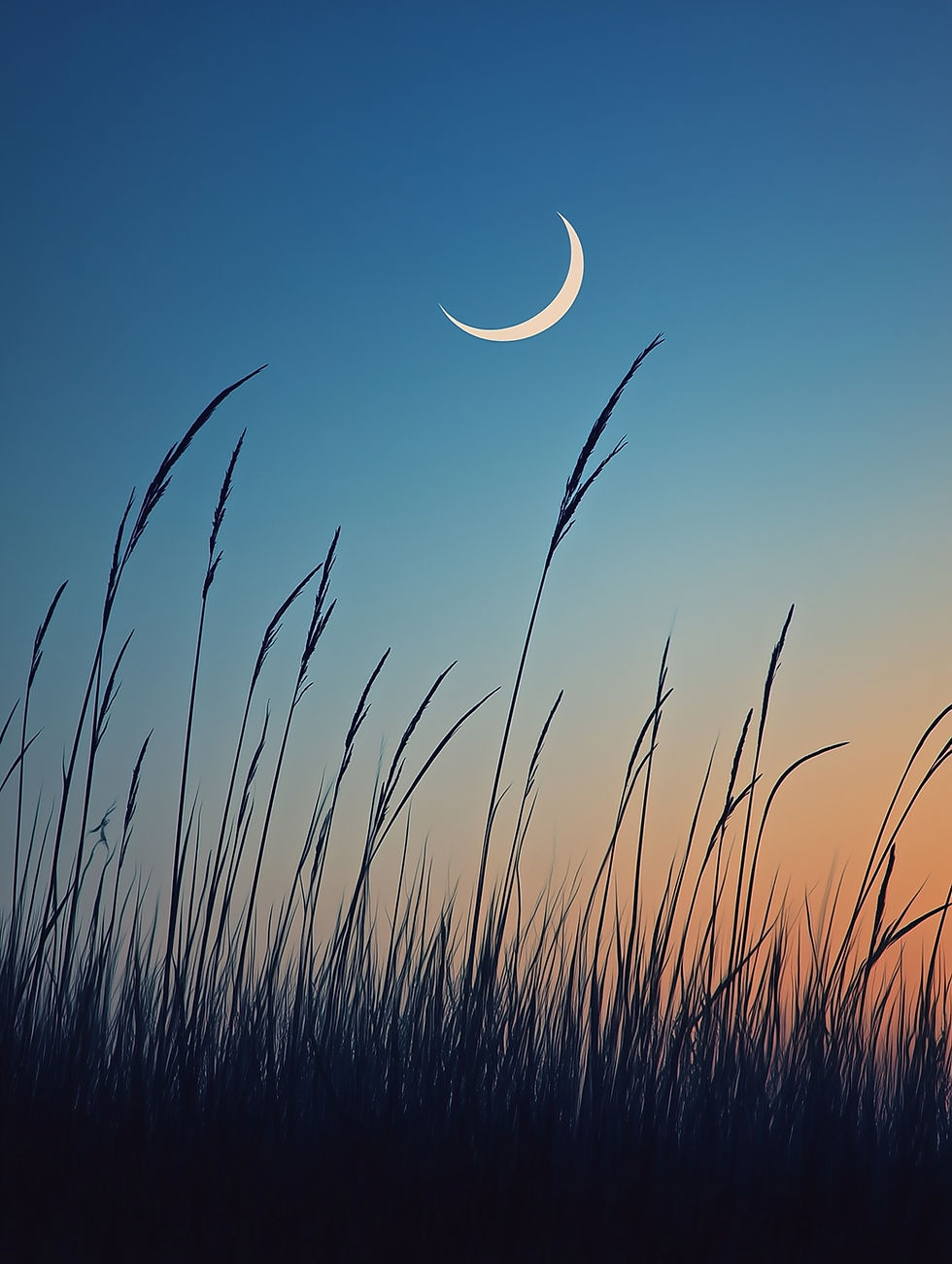
As many of us are nearing the end of another academic term, often we are asked to prepare a summary of our accomplishments for the year as part of a self-assessment program. Chances are most universities have clear guidelines and/or templates for creating and submitting these reports. Perhaps most of you have already been integrating AI into this process to aid in both efficiency and completeness. In either case, this week I would like to share a combination of articles and AI prompts which may be helpful to consider when organizing your annual scholarly accomplishments.
AI agents can prompt faculty to use broader measures of teaching effectiveness, including peer reviews, teaching portfolios, and learning outcome data (Berk, 2005). Faculty may be asked to triangulate data from several sources to create compelling teaching narratives. AI tools can support this process by helping faculty analyze and synthesize various data types, including:
Evidence Type | AI-Supported Application Example |
Student Ratings | Summarizing open-ended feedback or identifying thematic trends. |
Peer Ratings | Drafting response narratives reflecting on peer observations. |
Self-Evaluation | Assisting in reflective writing based on teaching goals and challenges. |
Video Analysis | Transcribing teaching videos and generating reflective prompts. |
Student Interviews | Organizing qualitative data and extracting representative quotes. |
Alumni Ratings | Synthesizing feedback over time to demonstrate long-term impact. |
Employer Ratings | Highlighting evidence of workplace readiness in students. |
Administrator Ratings | Aligning reflections with institutional teaching criteria. |
Teaching Scholarship | Generating annotated bibliographies of SoTL. |
Teaching Awards | Framing award narratives within broader teaching excellence claims. |
Learning Outcome Measures | Assisting in visualizing assessment data or crafting impact statements. |
Teaching Portfolios | Generating outlines and integrating multiple data sources cohesively. |
Faculty engaging in self-assessment can leverage AI to scaffold reflective practices. AI prompts can encourage deeper exploration of pedagogical approaches, such as using Brookfield’s (2017) four lenses:
self-reflection,
student feedback,
peer input, and
theoretical literature.
AI tools can generate potential narratives for how instructional strategies evolved in response to feedback or changing student needs, fostering growth-oriented language in teaching statements. Further, AI can assist in creating visualizations of teaching effectiveness data, identifying patterns in course evaluations, or generating text from rubrics or portfolios, making faculty self-assessments more efficient and comprehensive.
Although Student Evaluations of Teaching (SETs) are not the best method for assessing, measuring and evaluating effective teaching, they can be another data point to include in our portfolios [recall blog on Dec 14, 2018 on the bias of SETs: “There is no significant correlation between SETs and learning (Uttl, White, & Gonzalez, 2016); There is strong evidence that SETs do not measure teaching effectiveness (Stark & Freishtat, 2014); The measure of effectiveness is negatively correlated with SETs (Braga, Paccagnella & Pellizzari, 2014); and The relationship between gender and SETs indicate that the use is discriminatory against women (Mitchell & Martin, 2018)”].
As an additional data point, AI systems can assist by summarizing and coding SET data since these are often misinterpreted or overemphasized in annual reviews (Spooren, Brock, & Mortelmans, 2013). AI agents can contextualize these evaluations by analyzing trends within and across academic terms; and providing evidence-based strategies for improvement. An example prompt which you might use to analyze SET qualitative data might include:
“Analyze the following text for keyword extraction, sentiment analysis, thematic analysis, and clustering for [copy/paste the student comments from your SETs here]”
References
Berk, R. A. (2005). Survey of 12 strategies to measure teaching effectiveness. International Journal of Teaching and Learning in Higher Education, 17(1), 48-62. https://www.isetl.org/ijtlhe/pdf/IJTLHE8.pdf
Spooren, P., Brock, B., & Mortelmans, D. (2013). On the validity of student evaluation of teaching: The state of the art. Review of Educational Research, 83(4), 598-642. https://doi.org/10.3102/0034654313496870
Comments