Reinforcement Learning Scoping
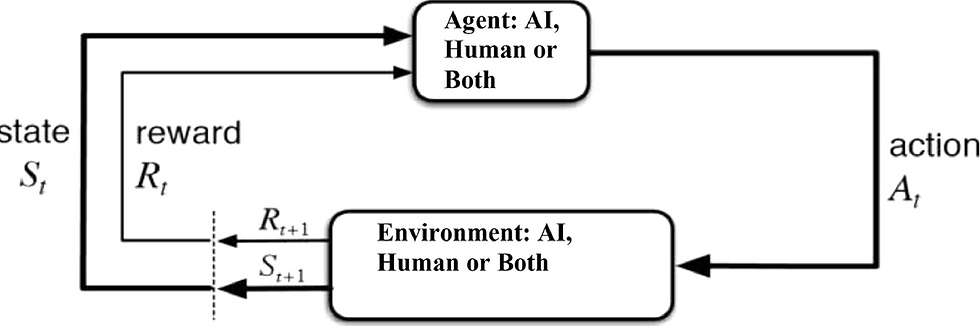
This week I would like to share an article which may not be directly considered SoTL, but I believe with the current updates in AI, this paper helps us connect foundational learning to aspects of AI training. The article is entitled, “A scoping review of reinforcement learning (RL) in education” by Memarian and Doleck (2024). As a reminder, a “scoping review” is a form of knowledge synthesis that addresses an exploratory research question aimed at mapping key concepts, types of evidence, and gaps in research related to a defined area or field by systematically searching, selecting, and synthesizing existing knowledge. The RQ for this study:
How are the characteristics of RL noted in the reviewed studies?
You may recall that I shared an article on RL about a year ago, “reinforcement indicates that the consequence of an action increases or decreases the likelihood of that action in the future. RL draws inspiration from various psychological theories, including operant conditioning. Conditioning is strengthening of behavior which results from reinforcement (Skinner, 1965). Some researchers are making connections between historical educational RL and Q-Learning (Watkins, 1989). Q-Learning is a RL policy that will find the next best action, given a current state. It chooses this action at random and aims to maximize the reward.”
The authors point out that the “use of AI and Machine Learning (ML) algorithms is surging in education. One of these methods, called Reinforcement Learning (RL) may be considered more general and less rigid by changing its learning through interactions with the environment. Given that education has shifted towards a constructivist approach, the authors conducted a scoping review of the literature on RL in education.”
Further, they “contend that education is reformed to adopt a socially constructive paradigm, enabling learners to construct knowledge through interaction based on their prior experiences. RL, however, is inherently rooted in a behaviorist paradigm, seeking punishments and rewards to shape learning.”
Key components of RL are known to be the:
States: Observations on the environment the agent can make upon acting in the environment;
Action: What an agent performs on the environment based on the state observations;
Reward: Feedback the environment provides to the agent to shape their actions. A reward can be either positive (rewards) or negative (punishment).
RL may be better understood through a preview of ML. Two key types of ML are supervised and unsupervised learning. Supervised learning is directed at how to learn from the environment primarily through labeled data sets. Unsupervised learning lacks labels on data sets. As such the unsupervised learning discovers how grouping needs to be done and predicts where each data set lies. There is a connection of this approach to the foundational teaching methods of inductive and deductive. Supervised learning aligns with inductive learning (learning general rules from specific examples). Unsupervised learning is less directly related to deductive learning, but it involves pattern discovery rather than rule application.
The findings of the scoping review on RL in education and AI identified only 15 focused studies. This highlights the necessity for further research. Overall, they found that RL is most frequently used in games, as they inherently depend on states and actions and feedback to the player.
References
Memarian, B., & Doleck, T. (2024). A scoping review of reinforcement learning in education. Computers and Education Open, 6, 100175, https://www.sciencedirect.com/science/article/pii/S2666557324000168
Comments